Our recent work from the IPA Fellowship was a new collaboration that allowed the firm to explore ideas about urban environments, infrastructure, and equity that have been central to our practice since the firm’s beginning. We have always been interested in how computational design could transform the practice of urban design and planning – both by diversifying the metrics used to develop physical form and economic value and to create new metrics by which planning outcomes are measured for success.
When we think of urban development, there is an existing framework of zoning that relies heavily on a few factors such as Floor Area Ratio, heights, yards, etc., that tend to drive physical form. While these can be complex at times, fundamentally they are a simplistic set of relationships that are a legacy of the past – derived from laws that were meant to address health and legal problems from nearly a century ago. Do they still work? Are they addressing the current needs of climate resiliency, inequity, open space? Are they even useful at all for the scale of planning we need? How can we begin to change them to be more responsive? And ultimately, can a new set of tools be put in the hands of communities and stakeholders to assess the possibilities and outcomes for themselves?
The existing framework of zoning is fundamentally a simplistic set of relationships that are a legacy of the past. Do they still work? Are they addressing the current needs of climate resiliency, inequity, open space? Are they even useful at all for the scale of planning we need?
We are only at the very beginning of our research into finding some answers to these questions and testing out computational tools that may give us some answers. But we’ve made some headway and have a few insights to share about what we’ve found so far and where we might be heading.

A generic set of city blocks first subdivided into parcels and assigned typical lot coverages as per NYC Zoning
The zoning resolution is very specific and was written for relatively small-scale development. What’s interesting is that within the code itself is the acknowledgement that the typical rules might not work well at a large scale – and so the zoning resolution allows for something called a large scale plan. At this scale, the resolution allows for waivers and all sorts of overrides if they aid in creating a better overall plan. Which is a good thing in a way. But how do you decide what’s a good overall plan? And who decides? Stripped of bulk controls, the city planner and the developer decide.
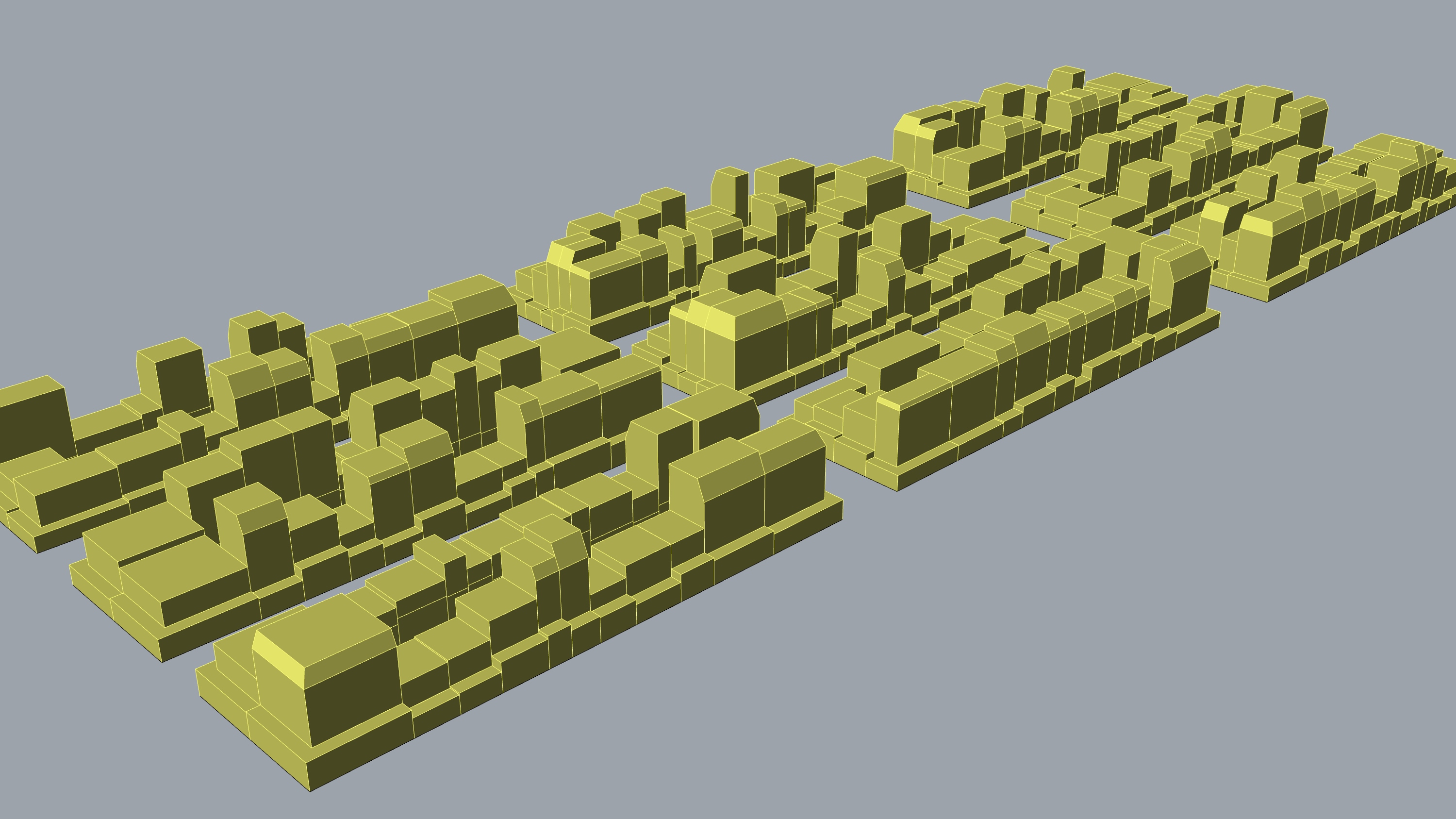
Massing generated for each parcel through FAR calculations and other bulk regulations
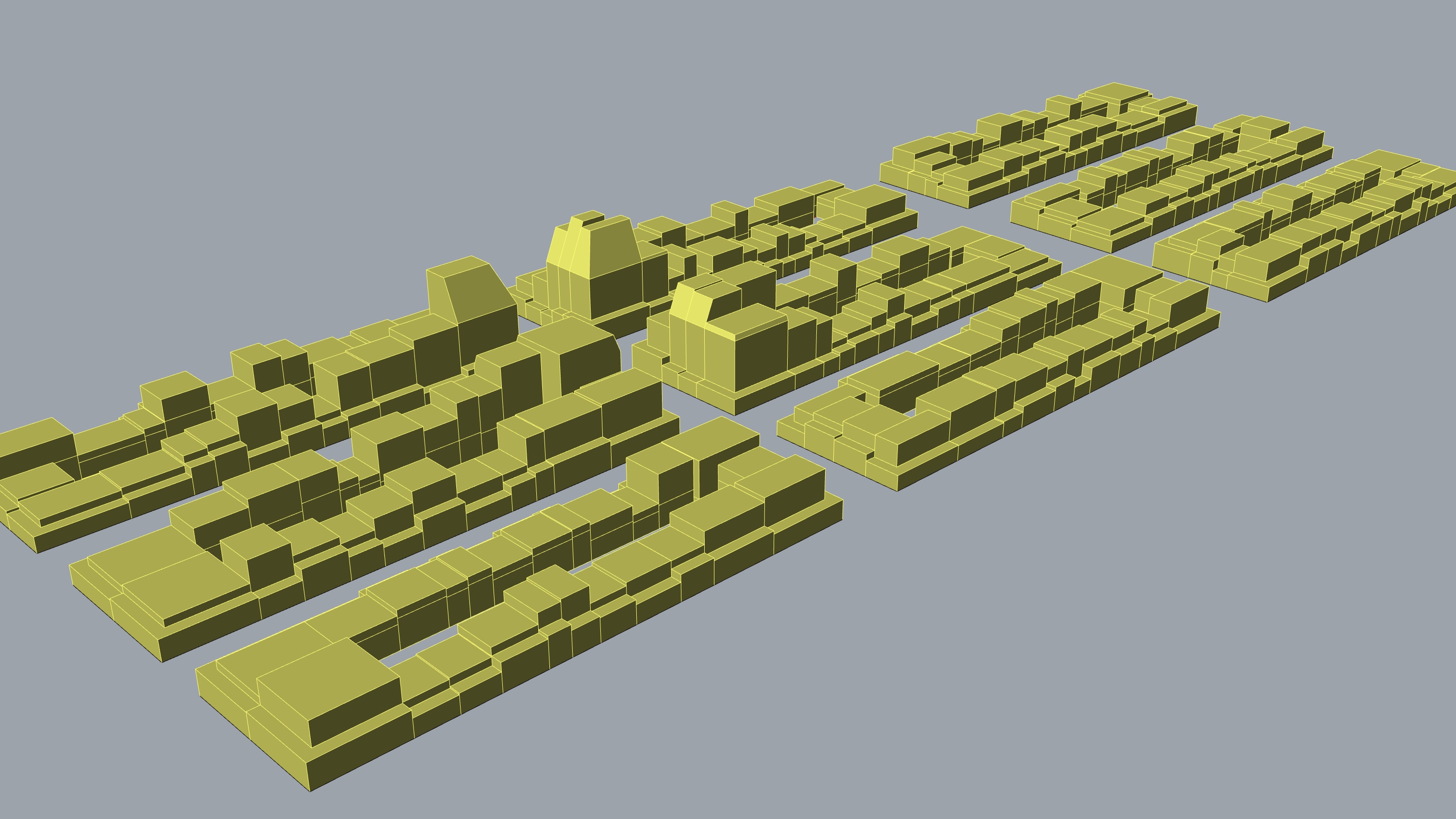
Modification of massing using a density hotspot to override generic bulk regulations
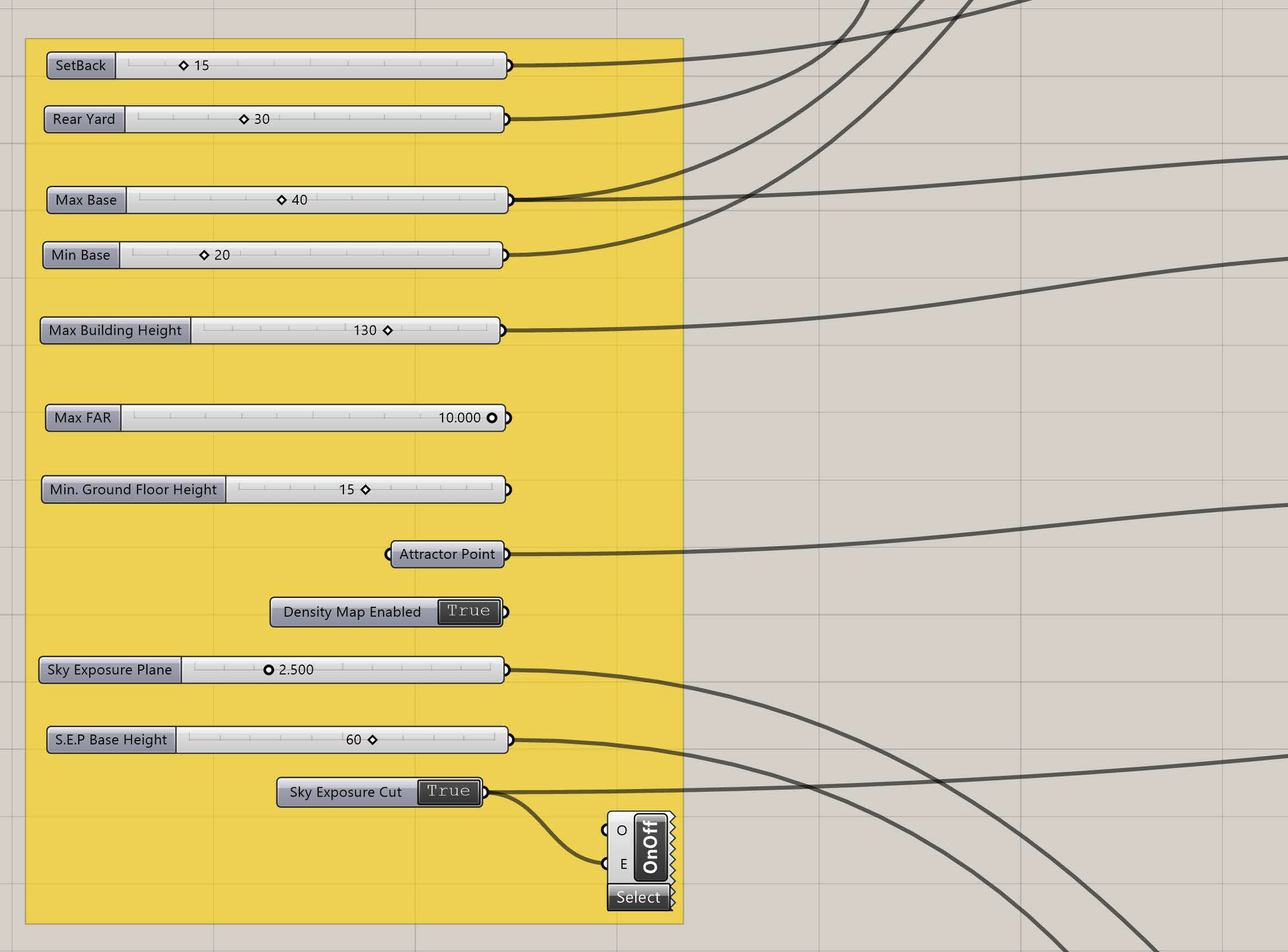
Detail of parametric tool that allows us to quickly explore the effect of bulk regulations at urban scale
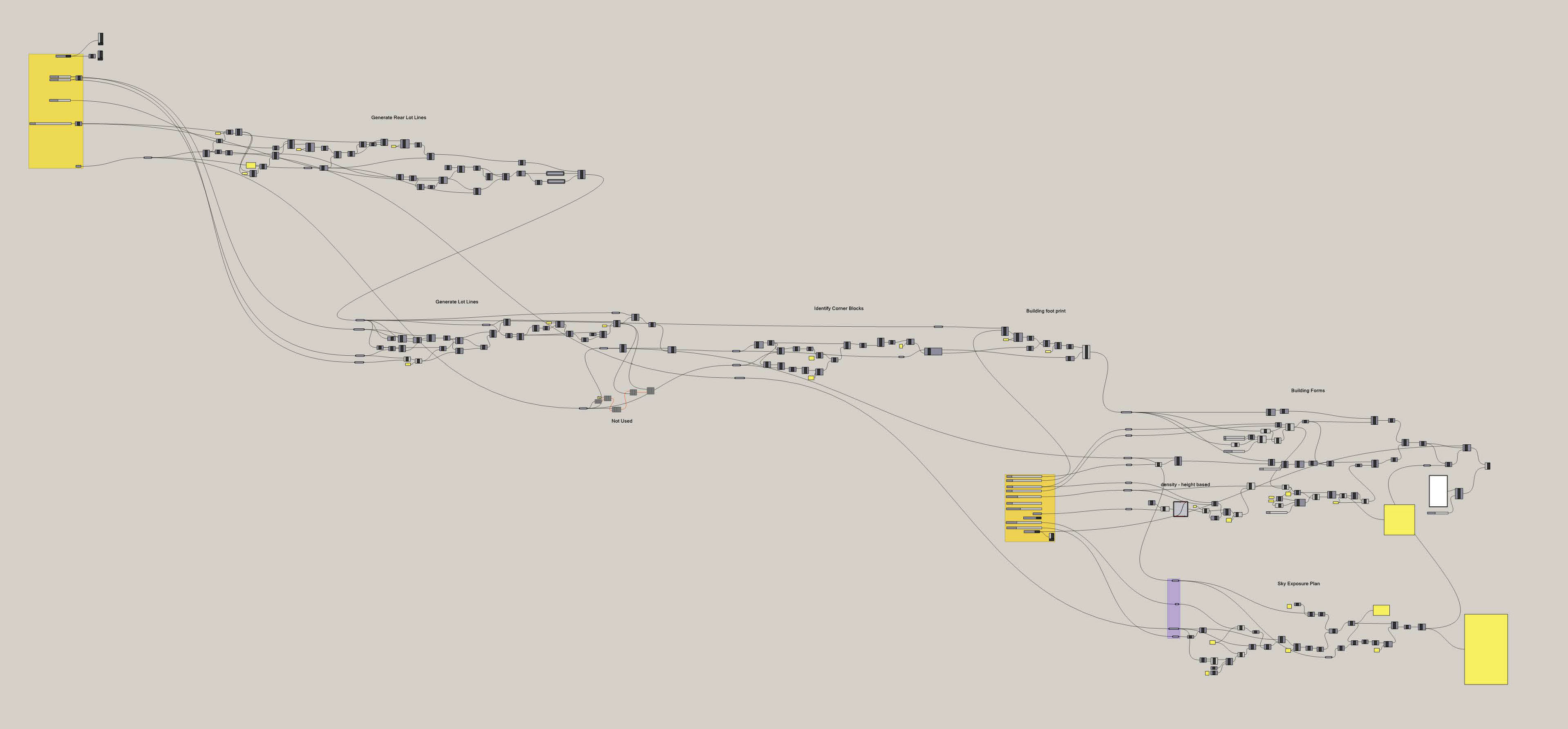
Schematic of our computational logic for exploring zoning metrics
If we can do that, what else can we add or tweak? What other metrics can we ask the zoning resolution to consider if it becomes more of a live tool? What we found in creating a tool to automate massing with the current set of zoning rules is that it was designed for rectangular parcels and the typical city grid. But what happens if we have a large undeveloped parcel with no existing street grid or lot divisions such as Willets Point or in our case, the Brooklyn waterfront along Piers 7 – 12?
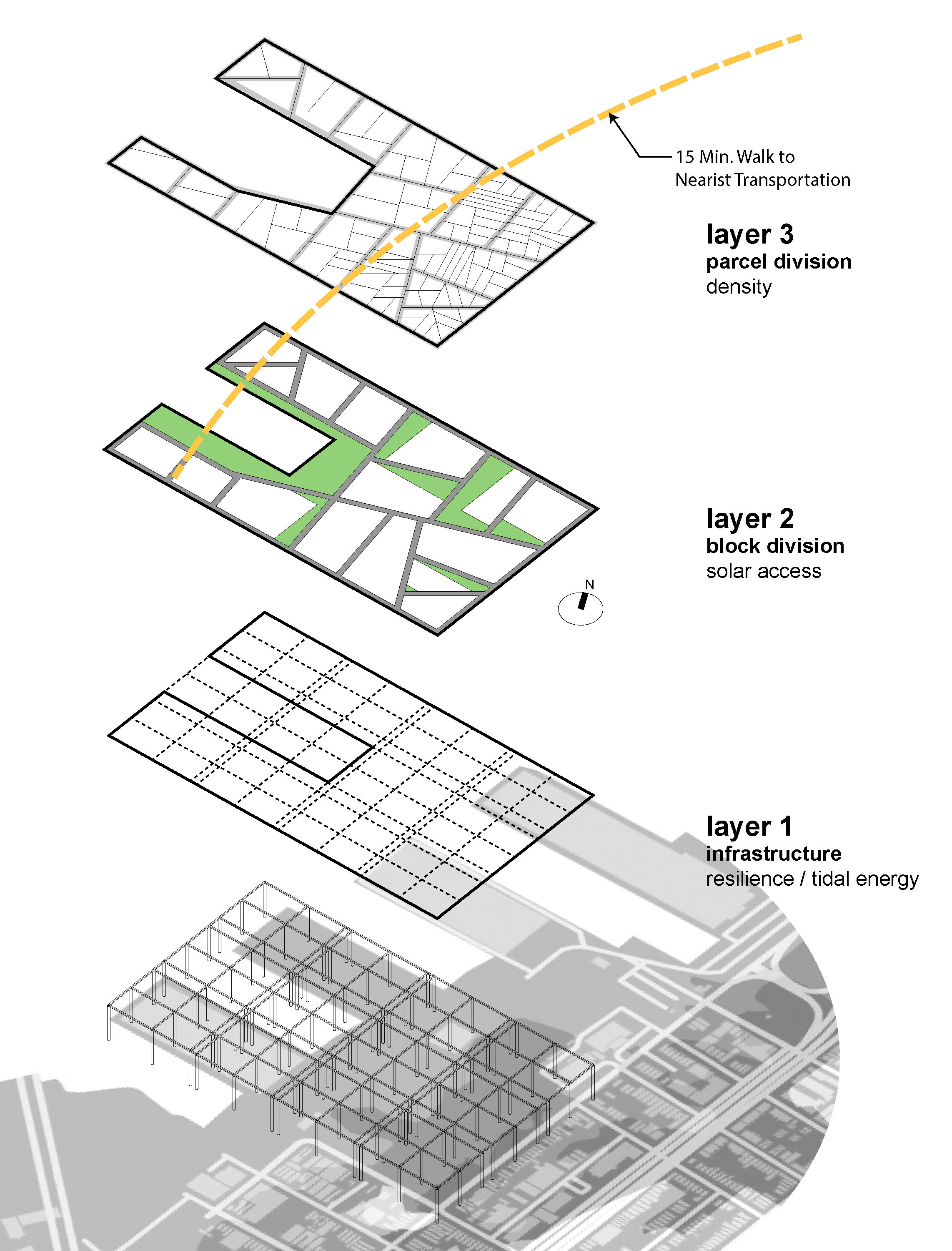
Should we assume an orthogonal grid? If so why? In the case of the piers, the projected sea level rise and storm surge are much more urgent concerns. What if the underlying structure of the urban plan for this area was driven by climate data? If we look at this area, open space and infrastructure should probably define the street grid and available land for development first. And of least importance is the shape and size of land parcels which can be variable depending on projected use.
In this way, we could build an analytical process that uses real-time data to integrate infrastructure planning into open space development and could lead to more vibrant neighborhoods with a mix of uses that are not siloed as they are under the current zoning.
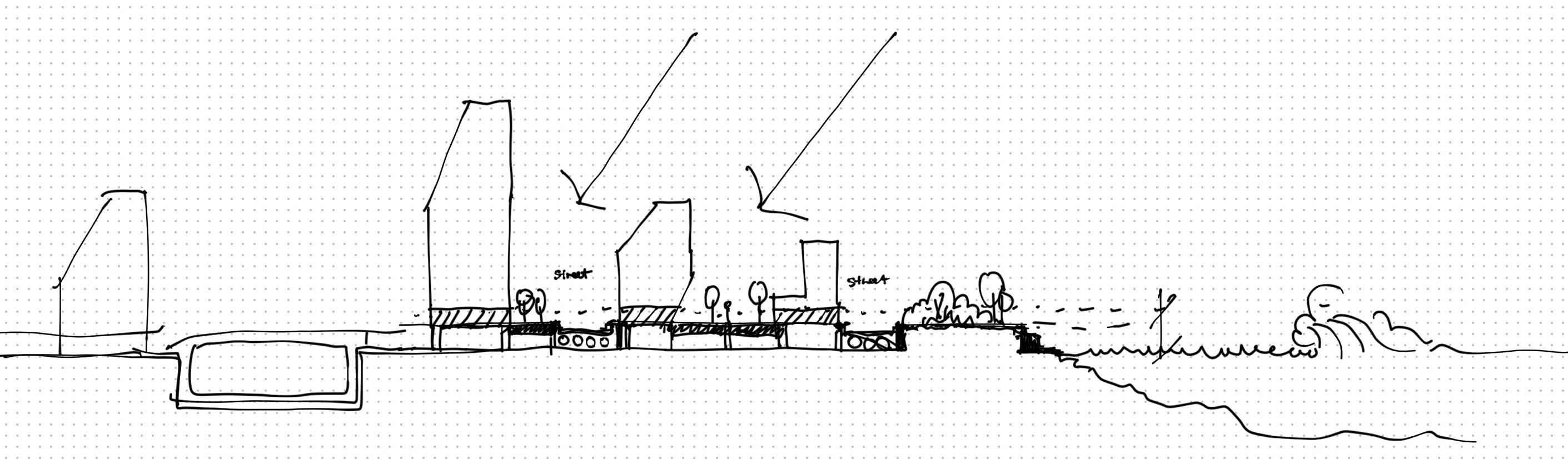
Concept section of potential development guided by new zoning and planning tools responsive to new metrics
At this point we started to get really interested in the possibilities. The breakthrough in the process outlined above was the ability of our tool to read color data from an image file and use it to run different processes – in this case a density gradient. However, the image data driving the parcel size and distribution was a topo map. But a topo map is not really useful information in terms of real underlying data that could inform real planning. So our next question was how do we take relevant and real-time locational data, visualize it as an image, and use that to drive our planning tools?
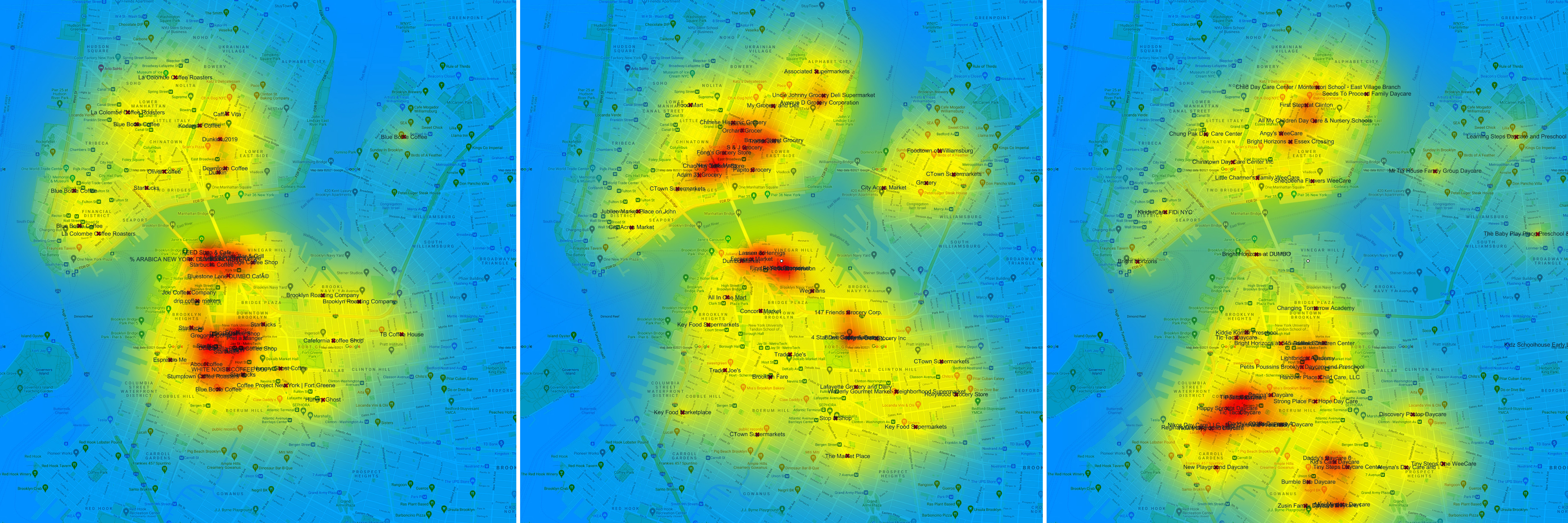
Data visualization of geo-located data from Google search results used to create "heat maps" showing gradients of density
And we are currently pulling real-time traffic data from Tom-Tom, GIS information from the city’s database, weather information, and other real-time data and are beginning to overlay all of this onto our base maps. The goal is to go back to our study of the Brooklyn waterfront and create a more intelligent set of tools that we can use to analyze this data and propose the right set of metrics. The more we explore, the more we realize that no single tool or process will get us to better planning outcomes. But these new tools can open our eyes to new data, new metrics, and new possibilities that ultimately need to be in the hands of communities and stakeholders so they can decide what the goals are.